Brian Malone, a writer for dynastyleaguefootball.com, has put together a great guest post today. You can follow him on Twitter at @julesdynasty. Thanks to Brian for today’s article!
Putting lipstick on the YPC pig
We all know that that yards per carry is, as Danny Tuccitto puts it, nearly a “bunkum stat” in terms of predictive power. Even as a descriptive tool, YPC is tolerable but unsatisfying. Matt Forte (4.12) and Chris Johnson (4.15) had nearly identical YPC in 2015, but their paths to these numbers were notably different. Forte rarely got stuffed behind the line of scrimmage, and he was well above average at posting four-yard gains. Johnson, in contrast, was a home run hitter, padding his YPC with runs longer than 20 yards.
Painting a better picture
We could supplement YPC with the standard deviation of a player’s runs. Or, as Jeff Levy suggests, we could include confidence intervals to define a player’s “true” YPC. Both supplements add useful information, but neither smacks the reader in the face with the contrast between Forte and Johnson. For that, we may need a visual.
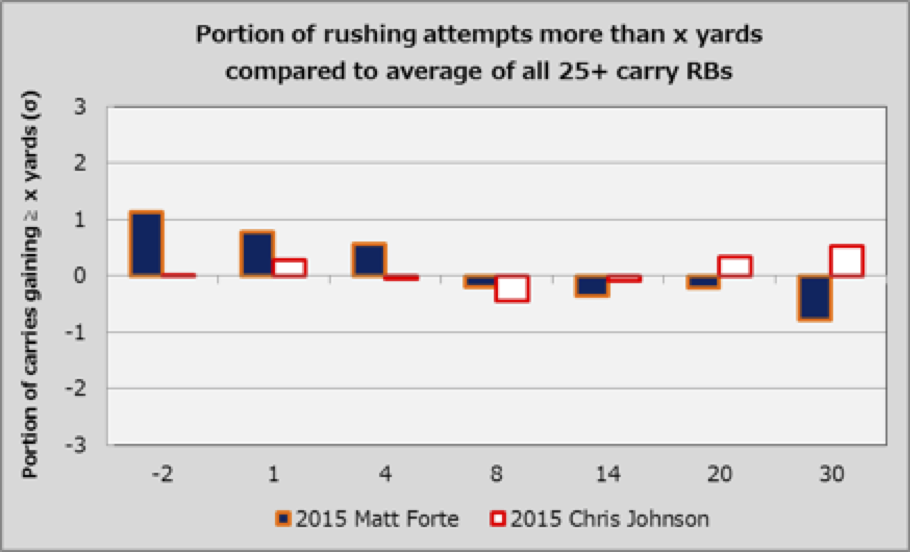
This shows what portion of a player’s runs went for at least x yards, compared to the average portion among all running backs with at least 25 carries in the same season. So Forte was roughly one standard deviation better than average at getting past -2, 1, and 4 yards, but average to below average at any distance beyond 8 yards. Johnson, on the other hand, was middling across the board. His biggest strength was a half-standard deviation advantage in breaking long runs.
One of 2015’s biggest home run hitters was Todd Gurley (4.83 YPC). He didn’t have a “YPC twin” like Forte and Johnson, but a quick search showed that Alfred Morris – decidedly not a home run hitter – put up 4.81 YPC in 2012. Here’s how they each posted those numbers:

Gurley was met in the backfield often, but this also suggests he wasn’t very successful getting through the second level of the defense. He made hay on long runs, but relying on those big gains is a dangerous proposition.
Gurley’s struggles behind the line of scrimmage prompted me to look at fellow freshman Melvin Gordon (3.48 YPC). Contrary to the “Chargers don’t have an offensive line” narrative, Gordon wasn’t obviously worse than Gurley at getting a few yards past the line of scrimmage. Gordon’s low YPC stems from his failure to break off runs longer than 4 yards.
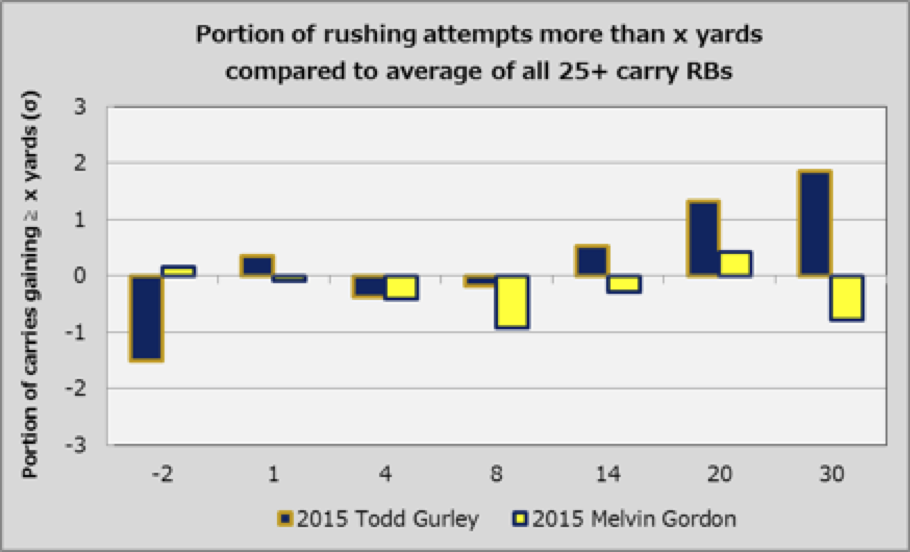
Looking at runs less than 8 yards, it’s tough to tell between Gordon and Gurley. But Gurley turned those 4-yard runs into 8-, 14-, 20-, and 30-yard runs at a much better rate.
Building a better metric
Now that we’ve created something that illustrates rushing production better than YPC, maybe we can use it to predict rushing production better than YPC. It’s a low bar, after all.
Indeed, we can clear the YPC bar, but maybe not by much. Using data from 2000 to 2009 and the buckets included in the above visualizations, I data snooped my way to a model [1]Without getting too deep into the weeds, the model assigns the following weights to standard deviations from the mean of the portion of a player’s runs gaining at least x yards: http://www.footballperspective.com/wp-content/uploads/2016/03/Screen-Shot-2016-03-23-at-7.36.04-AM.png 550w" sizes="(max-width: 300px) 100vw, 300px" /> that predicted season n+1 YPC better than season n YPC does (for running backs with at least 100 carries in each season). I then tested that model on 2010 to 2014 data, and the results were positive. Indeed, the model actually improved when applied to the testing data. Here’s the results across all seasons (n = 2000-2014).
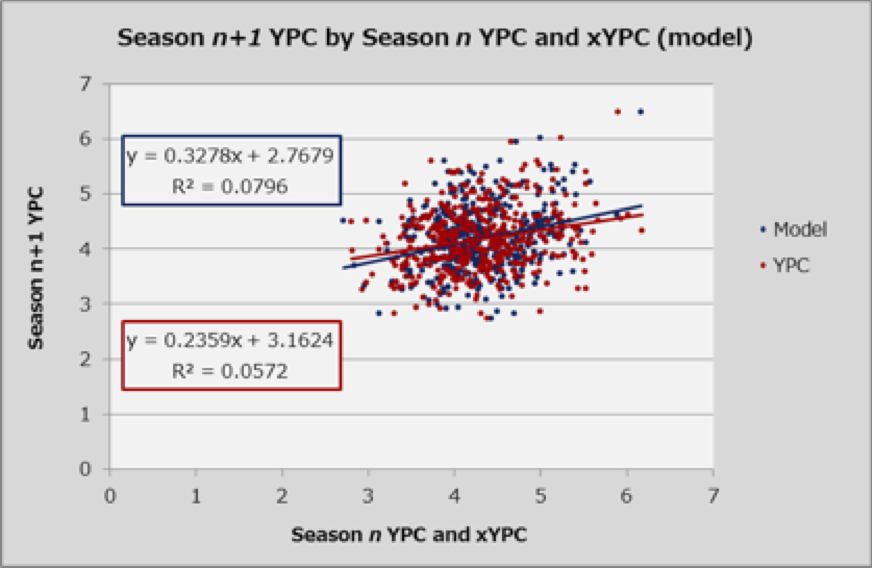
Nothing groundbreaking here. Each full YPC predicts an extra 0.24 YPC the following season, while each full xYPC predicts an extra 0.33 YPC the following season. The good news is we can probably build a better model than this one. My main goal was to illustrate running back performance; any predictive power is a bonus.
Speculating a better speculation
Even if this model isn’t optimal, I can’t not use it. Let’s see what it predicts for 2016.
The model doesn’t like Gurley (4.8 YPC; 4.2 xYPC) or Lamar Miller (4.5 YPC; 4.1 xYPC). That’s probably not a good sign for the model. For what it’s worth, Lamar Miller’s 2015 looks much like, say, Charcandrick West’s (4.0 YPC; 4.1 xYPC):
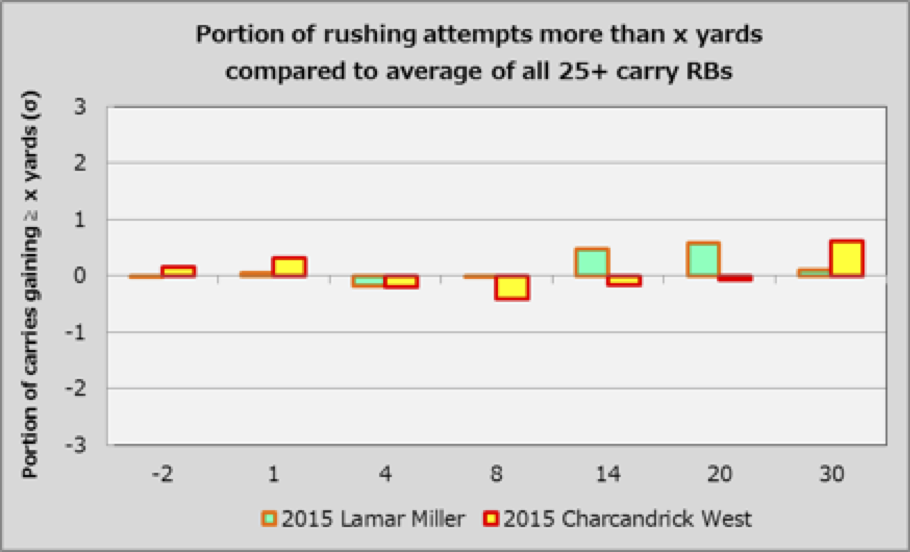
The model prefers mostly low-sample rushers. Perhaps most interestingly, it likes Forte (4.1 YPC; 4.4 xYPC), Jeremy Langford (3.6 YPC; 4.2 xYPC), and Ka’Deem Carey (3.7 YPC; 4.5 xYPC). That oddity prompted me to make one more visualization. I’ll let you decide how to interpret it.
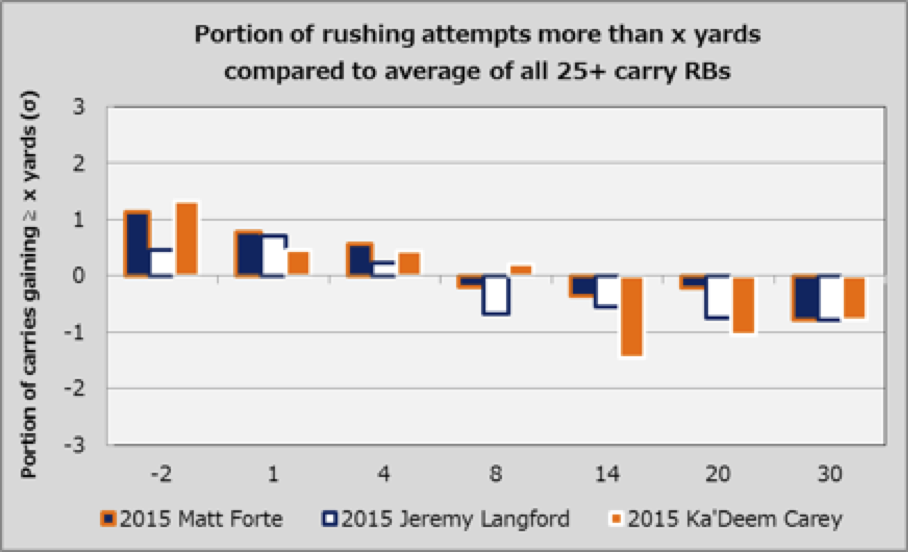
References
↑1 | Without getting too deep into the weeds, the model assigns the following weights to standard deviations from the mean of the portion of a player’s runs gaining at least x yards: ![]() |
---|